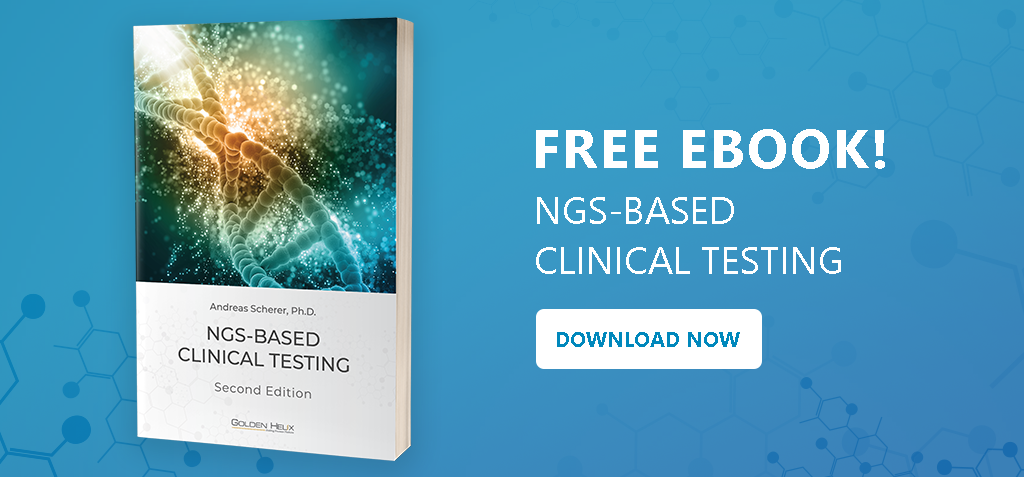
After the Wet Lab process has been completed, the bioinformatics analysis of the sequencing data work begins. The next three blogs will focus on three aspects of this process.
- The building blocks of a bioinformatics pipeline, documentation and validation (today’s topic)
- Quality Management
- Clinical Reporting
The Building Blocks of an NGS Pipeline
The bioinformatics process to analyze NGS data occurs in three steps. First, we need to generate the sequence read file. This consists of a linear nucleotide sequence (e.g. ACTGGCA), with each nucleotide being assigned a numerical value that relates to its predicted accuracy. This step occurs within a DNA-sequencer, which is commercially available from companies such as Illumina. All sequence reads are stored in a FASTQ file generated from the sequencer. FASTQ files contain the compilation of individual sequence reads that are between 50 to 150 base pairs long. Depending on the selected coverage, a FASTQ file might contain millions to even billions of short read sequences. Generating the FASTQ file is also called “Primary Analysis”.
Second, the sequences in the FASTQ file need to be aligned vis a vis the human genome reference sequence. This is computationally an expensive step that alone cannot be solved optimally within a reasonable time frame. The underlying computer science problem of aligning reads to the reference sequence is NP-complete. Hence, there are many different types of algorithms in the literature described with different optimization goals. One of the standard aligners used in day to day practice is the BWA aligner. The output of this step is a BAM file which contains all the reads from the FASTQ file aligned to the reference. The next step in the process is to identify the differences between the patient sequence reads and the reference sequence. These differences might entail single nucleotide variations (SNVs) including insertions and/or deletions, copy number variations (CNVs) and other structural variations. There are a number of software tools available. For example, the Broad Institute has a widely adopted variant caller, called GATK. GATK can be used for germline mutations. There is a special version available that is being used to analyze cancer related mutations. This step of the bioinformatics process is referred to as “Secondary Analysis”. The result of this step is a VCF file that contains all identified variants of the patient sample.
Third, we need to enrich the VCF file by annotating all of the variants based on information from public and private data sources. Essentially, this step interprets the patient sample by identifying variants that are damaging or functionally impacting one or multiple genes, relevant to the patient’s observed phenotype. The number of variants in a VCF file depends very much on the initial target region (e.g. gene panels, exome or genome sequences). It can range from hundreds of thousands to millions of variants. In order to reduce the number of variants to only those that have high clinical relevance, a combination of quality filters, population frequency data and functional predictions are typically used. In the case of exome or genome sequences within a family, we eliminate variants that are present in the unaffected family members. Based on the resulting set of variants, it is possible to conduct a variant prioritization. This step utilizes public and private databases, such as Online Mendelian Inheritance in Man (OMIM), to identify the variants that are known to be associated with disease. The steps outlined to annotate, filter and prioritize variants is often referred to as “Tertiary Analysis”.
Documentation
Laboratories are obligated to document all algorithms, software and databases used in the analysis, interpretation and reporting of NGS results. The overarching objective is to create a repeatable pipeline that creates consistent results. This is a tall order. In reality, this means that each version of all pipeline components must be described and recorded. The documentation of each component may include a baseline, default installation settings and the description of any customization by using different configuration parameters, running different algorithms or deploying custom code…
To continue reading, I invite you to download a complimentary copy of this eBook below: